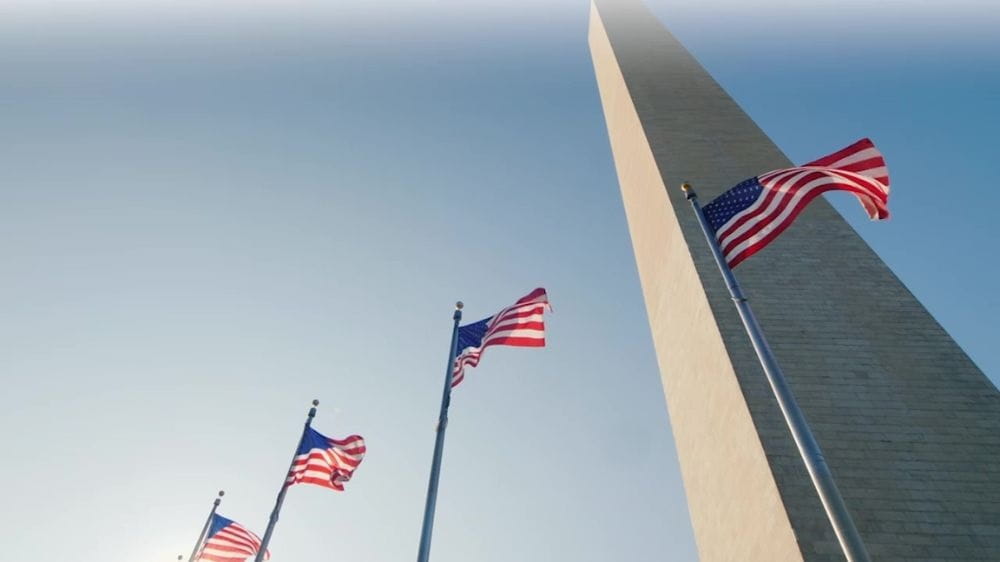
Hogan Lovells 2024 Election Impact and Congressional Outlook Report
Generative AI continues to make waves across industries in 2024 and the fashion and retail industry is no exception. Retailers are taking advantage of generative AI technology to develop smarter and adaptable virtual shopping assistants that can understand and interpret human language with greater accuracy, which unlocks the potential for chatbots to handle a wider array of tasks and to provide a more comprehensive and personalized online shopping experience.
The chatbots of the past are less flexible in the type and scope of assistance they can provide. Such chatbots are rule-based, meaning that these bots are taught to answer a defined universe of questions that are manually pre-programmed and to use simple keywords to find patterns in the customer’s questions to account for deviations in how sentences are phrased. While these chatbots can be helpful with a specific set of queries, the customer experience is stunted by the chatbot’s limited capability to engage beyond its pre-defined script.
Personalization is the name of the game when it comes to this new generation of chatbots. The latest rollouts of generative AI-powered bots use large language models that train on datasets, including customers’ searches, purchase history, and feedback, and can perform natural language processing tasks, like sentiment analysis, text generation, and translation, to understand the intent and context of the customer’s queries. Such advancements allow the bots to act like shopping assistants, who can provide more personalized responses and recommendations in a conversation-like dialogue, mimicking the dynamic interaction a customer would have with a sales associate in a brick and mortar store.
Luxury retailers, online marketplaces, and even payment technology companies are leveraging this new technology to elevate the online shopping experience by making the search process more intuitive.
Because the generative AI-powered shopping assistants can be trained on a retailer’s extensive product catalogs, customer information, and data from across the internet, shopping assistants are equipped to answer questions about specific products, research product categories, compare prices, and offer recommendations for specific occasions and use cases. For example, a customer could provide a range of prompts—such as “what are good gifts for coworkers,” “hosting Superbowl party,” “considerations for buying a new car,” or even modern trends like “balletcore”—and receive cross-category results that can be further fine-tuned through additional parameters and requests. Luxury fashion houses are also experimenting with AI personal shoppers that would provide recommendations for niche and selective luxury goods from a house’s family of brands for the more discerning repeat consumer. Payment processing companies also want a piece of the action by creating their own marketplaces, shopping assistants, and technology solutions, instead of simply being third party providers to retailers. Certain payment processing companies, like many of its e-commerce competitors, now allow customers to scan bar codes of products in brick and mortar stores to search for customer reviews, inventory information, and product details; to search using images and find online options for products they come across in day-to-day life; and to access a shopping assistant who can make the use of these features seamless from research to checkout.
A benefit of using large language models to power these shopping assistants is that the assistants are trained to adapt to an individual consumer’s unique profile, affinity, and colloquialisms using large sets of data over time to deliver tailored recommendations without the need to fill out the interminable questionnaires that the previous generation of personalized online shopping experiences required. By expanding the search capabilities in a one-stop shop, customers can avoid the endless scrolling that comes with e-commerce, and retailers can engage and influence customers even earlier in their shopping experience—when they are still researching their options and are open to recommendations—which could boost conversion rates and sales. The new technology also collapses the experiences between online shopping and in-store shopping, by providing customers the searchability of the online shopping experience in brick and mortar stores while potentially deepening the relationship between customer and retailer.
As more and more retailers adopt generative AI-powered shopping assistants to maintain a competitive edge, we can expect to see retailers expand their AI bot capabilities, through building custom features in house, acquiring existing AI companies, or by partnering with startups or platforms that offer development frameworks to create chatbots. At the recent Silicon Valley M&A Forum, a tech investment banker on the panel stated that deals involving generative AI companies consisted of only 5% of the M&A landscape before the introduction of ChatGPT, but that percentage has since skyrocketed to 20% in less than two years and will continue to grow as more funding and attention is being invested in nurturing AI talent. With the increasing demand for virtual shopping assistants and generative-AI offerings in general, we will see a flood of new startups offering chatbot tools and infrastructure to accommodate retailers of various sizes, industries, budgets, and scalability. Technology giants have also begun to develop their own platforms for its existing and potential enterprise customers, which helps the companies cross-market a suite of products.
However, the new technology is not without its limitations. The search results and product details generated by the shopping assistants could include inaccurate or outdated information that are scraped from unverified sources on the internet. There is also a concern that non-public and personal data could be used to train the chatbots. Not only do these concerns weaken customer trust and goodwill, but they could lead to publishing unlawful or defamatory speech, violating data privacy rights, or misappropriating intellectual property rights, which may expose retailers to lawsuits.
Several cases regarding the legitimacy and governance of AI-generated content are currently pending in federal courts, most of which concern copyright infringement claims. Most recently, in Gonzales v. Gonzales, the U.S. Supreme Court considered the question of whether algorithmic recommendations should receive the full legal protections of U.S. Code Section 230, which shields internet platforms, including online marketplaces, from being held responsible for the material posted on their sites. Although the case largely applies to user-generated content for platforms such as traditional search engines, the Supreme Court’s decision may have implications on the future of AI-generated chatbots and search features and whether such interfaces can rely on the Section 230 safe harbor protections if they are not merely linking or repeating material (as in the case of many traditional search engines) but instead using predictive algorithms to edit or summarize third-party material.
On March 18, 2024, the Supreme Court came down with an opinion that sidestepped the issue for now and rejected efforts to limit the scope of the protections that Section 230 creates for the technology platforms. The decision is touted as a temporary victory for technologists, who remain free to develop and innovate new uses for generative AI without restraint from legal precedence, but the laws are far from settled. In the meantime, the adoption of generative AI-powered shopping assistants will become increasingly prevalent and inevitably shaped by the legal landscape as the law, like the technology it governs, continues to adapt.
Authored by Angela He.